
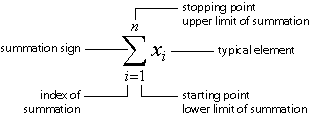
The implications of this selection bias are quantified, and a simple bias correction factor is introduced. Using 1 minimal assumption about the parameterization of the selection function, it is shown that unless sampling of species is random, the distribution from which toxicity data are actually sampled is never the distribution that is assumed. Before looking at the implications of small sample sizes and selection bias on the estimated hazardous concentration, the strengths and weaknesses of the SSD approach are reviewed. Not surprisingly, this has profound implications for both the fitted SSD and the derived hazardous concentration.
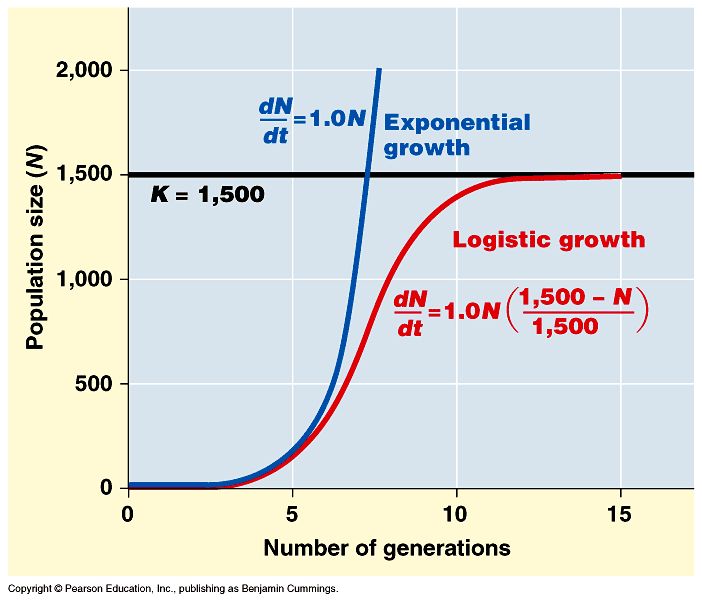
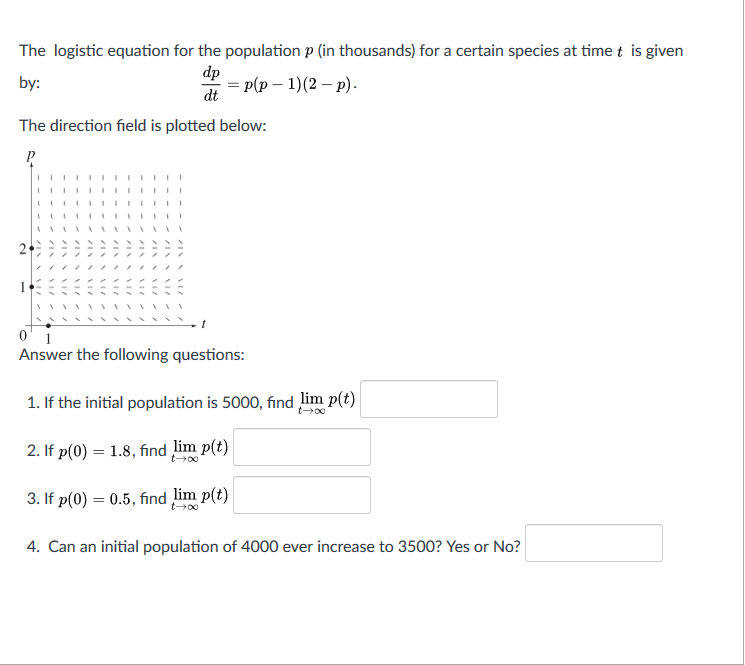
Importantly, it is demonstrated that toxicity data assumed to be randomly generated from an underlying log-logistic distribution in fact represent a sample from an F distribution when selection bias is present. They have been derived from mathematical and statistical considerations using models and assumptions that reflect actual ecotoxicological practice. The results of the present study are not based on any specific data set(s), simulated or otherwise. Although the ease with which this form of analysis can be undertaken is appealing, it has a serious limitation-the inability to unequivocally generalize findings beyond the individual data sets and parameterizations used. For reasons of analytical tractability and/or convenience, simulation methods are often used to explore SSD modeling assumptions. The choice of the statistical method” ), it is generally ignored in practice. # 2015 SETAC Keywords: Species sensitivity distribution Importantly, if the toxicity data are biased toward the more sensitive species, then the conventional practice of using the lower confidence interval limit for the estimated hazardous concentration may be compensating in the wrong direction. It is shown in the present study that, under very minimal assumptions, nonrandom species selection can result in hazardous concentration estimation errors of a factor of 20 or more. Although universally recognized as a crucial prerequisite for the statistical validity of the procedure, the assumption of random selection of species for SSD modeling is invariably violated. FOX*yz yEnvironmetrics Australia, Beaumaris, Victoria, Australia zUniversity of Melbourne, Parkville, Victoria, Australia (Submitted 1 April 2015 Returned for Revision Accepted ) Abstract: The species sensitivity distribution (SSD) has been an important development in ecotoxicology, and despite numerous concerns having been raised over many years, it remains the preferred (and often mandated) technique for establishing “safe” concentrations of contaminants in receiving water bodies by jurisdictions around the world. SELECTION BIAS CORRECTION FOR SPECIES SENSITIVITY DISTRIBUTION MODELING AND HAZARDOUS CONCENTRATION ESTIMATION DAVID R.
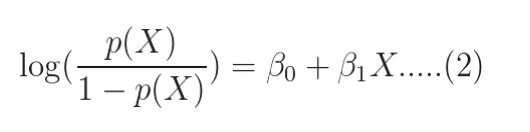
Run-time complexity for this model will be O(d), so this is very useful for low latency application.Environmental Toxicology and Chemistry, Vol. Space-time complexity for this model will be O(d) as we only have to store weight matrix for prediction Space-time complexity of Logistic RegressionĪt training time we have to go through every data points so train time complexity is O(nd) Assumptions of Logistic RegressionĪll the above will be possible only if we make below assumptions. If we find the absolute value of each feature and sort them descending, then the most important features will be at the top. We have weight W after training the model. Logistic regression is highly interpretable. Note that our model will be more confident in predicting class if the value of W.TXq is large (If the value is closer to 0 then the confidence of the model will low)
